I recently came across ‘Can Data Scientists spell Sustainability?’ by Yogi Schulz
The article portrays the opposing views held by data science and information system departments and how each side view their responsibilities: “Data scientists see only throw-away software that will be discarded once the breakthrough insights have been actioned by management. The [information systems] department sees only thoroughly designed and tested software that will be used in production-quality applications.”
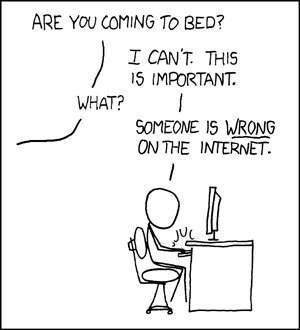
While I acknowledge the challenges, I don’t think that pinning data science against information system (technology services) departments, or as presented, sustainability against flexibility, tells the full story.
I personally can’t imagine a situation in which data scientists at Netflix, YouTube or Tesla are blamed for not delivering on sustainability or for their ‘information systems’ counterparts are blamed for being rigid.
Data science projects
In a nutshell, data science projects are in essence, solutions development projects. Data scientists use data, tools and code to deliver a digital solution. Those solutions are, in turn, used to help make better business decisions or optimize certain functions.
They can be as simple as a stand-alone script that generates frequent reports. Or as complex as a multi-year, multi-million dollar solution intended to create a self-driving vehicle.

From a personnel perspective, data science projects are cross functional and involve multiple roles. On a smaller scale, those roles can be assumed by a single person (a data scientist/unicorn?). On a larger scale, it’s a cross functional team with business owners, product owners, data scientists, front-end developers, back-end developers, data engineers, and more.
From a solutions perspective, data science project results and outcomes must be seamlessly embedded within business workflows. Data science project results that are not easily used in making business decisions remain largely experimental and gimmicky.
Technology Services
So – why does the introduction or growth of data science practices feel challenging? Why do data scientists feel constrained and perhaps isolated with push back from their technology services departments?
In my opinion, this might just be a secondary symptom of how less-equipped technology services departments have become.
Many technology services departments have chosen, or were forced, to become cost centers that are responsible for commodity hardware or off-the-shelf services, not the business partners needed to move the business forward.
In the process of standardizing their services, some might have shed their technical talent in favor of managerial or business one.
Their ability to develop and implement technical solutions ended up with third parties at the expense of adaptability and agility and with costs that far exceed the effort.
In this type of environment, data scientists with their need to develop technical solutions or integrate their work in the larger technological landscape, face repeated pushbacks.
Access to complementing tools or talent become entangled in process and budgetary discussions, and simple technical requests become missions to mars. A ripe environment for Shadow IT.

Data Science within Non-Tech Organizations
I would like to think of the challenge as an organizational responsibility rather than an A-against-B argument.
Organizations serious about adopting data science as a competitive advantage, or even as an investment attractor, should also consider themselves as technology creators rather than just technology consumers.
Some of the things that organizations can do to promote and encourage the development of technical solutions (rather than ‘data-science projects’) are:
- Promote and encourage ways to properly deliver digital solutions, rather than just isolated ‘data-science projects’.
- Embrace principles that value people, collaboration, flexibility and working solutions.
- Adopt processes to manage knowledge projects as well as minimize the risk during delivery.
- Implement methodologies to better understand the target [internal] market and measure traction.
- Develop technical capabilities to improve their overall competitiveness and offer services or products unmatched by their peers.
For many years, those principles, strategies, processes and methodologies have been battle-tested at technically inclined organizations. What it took is a realization, direction, learning and will.
Acknowledgment
Thanks to Brett Wiens and Marc Boulet for the first read and feedback!